Illicit financial flows
Measuring illicit financial flows for stronger domestic resources
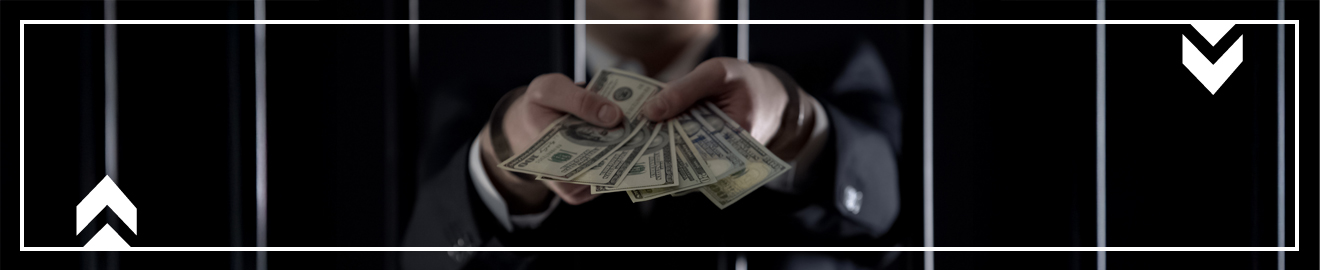
The 2030 Agenda identifies the reduction of IFFsIllicit financial flows as a priority, as reflected in target 16.4. This target is essential not only for financing the SDGsSustainable Development Goals but also for ensuring economic sustainability. After global agreement on concepts and methods1, the first official estimates of IFFs were reported in 2023 revealing alarming volumes of funds crossing countries’ borders due to criminal activities.2 In addition, experimental estimates of tax and commercial IFFs also reveal significant flows, ranging from 5 to 30% of official goods trade in many African pilot countries. For instance, in Namibia, such flows were estimated to exceed 8% of GDPGross domestic product (GDP) is an aggregate measure of production, income and expenditure of an economy. As a production measure, it represents the gross value added, i.e., the output net of intermediate consumption, achieved by all resident units engaged in production, plus any taxes less subsidies on products not included in the value of output. As an income measure, it represents the sum of primary incomes (gross wages and entrepreneurial income) distributed by resident producers, plus taxes less subsidies on production and imports. As an expenditure measure, it depicts the sum of expenditure on final consumption, gross capital formation (i.e., investment, changes in inventories, and acquisitions less disposals of valuables) and exports after deduction of imports -—
– ‒
- –
—--—
– ‒
- –
—--—
– ‒
- –
—--—
– ‒
- –
—-. in 2022.3 If redirected into the formal economy, these illicit flows could serve as a vital source of funding for sustainable development, helping to bridge the financing gap -—
– ‒
- –
—--—
– ‒
- –
—--—
– ‒
- –
—--—
– ‒
- –
—- and limiting the impact of the destabilizing international development assistance crisis.
Recognizing this, the UN General Assembly welcomed the first official estimates of IFFs based on internationally endorsed methodology. It called on co-custodian agencies, UNCTAD and UNODCUnited Nations Office on Drugs and Crime, to strengthen support for member States, especially developing economies, by providing technical guidance and tools for data compilation and reporting, and invited all member States and international organizations to engage with the custodian agencies for more informed and effective policy action and reporting of data on SDGSustainable Development Goal indicator 16.4.1 -—
– ‒
- –
—--—
– ‒
- –
—--—
– ‒
- –
—--—
– ‒
- –
—-.
Source: UNCTAD and UNODC.
Note: Situation reflected on the map as of April 2025.
To date, 22 countries have piloted IFFIllicit financial flow measurement. Among them, 14 countries (12 in Africa and two in Asia) have tested methods to measure tax and commercial IFFs while nine countries have tested measuring IFFs from illegal market and exploitation activities.
Towards Agenda 2030: closing country data gaps
Over the years, UNCTAD and partners have trained over 2 000 experts in analysing tax, customs, and banking transactions to identify sings of illicit finance. Dialogue between statisticians and policy makers is key to effective response. To this end, UNCTAD hosted a Joint Measurement and Policy Workshop6 in February 2025 in Geneva, bringing together 30 experts, including 13 women, from Ghana, Namibia, and Zambia and academic and research institutes. Findings shared at the seminar, illustrated high IFF risks related to the trade of raw minerals, like gold, manganese, copper, and uranium, and crude oil, as well as agricultural produce. Some countries revealed significant under-invoicing for certain critical minerals, such as copper or cobalt.
The experience of these countries shows that inter-agency collaboration is key in tracking and fighting IFFs. Institutionalizing platforms such as national coordination mechanisms, technical working groups, or establishing a dedicated IFF unit, strengthens both the measurement process and the broader governance of IFF data. These structures enhance transparency and help address persistent challenges, such as lack of data-sharing agreements and confidentiality constraints, as well as technical issues related to data coverage, quality, and interoperability. Building the capacity of key staff is crucial for effective tracking and curbing of IFFs. Equally important are the adoption of global standards for IFF reporting and reinforcing legal and institutional frameworks at the national level. Together these efforts support both the measurement and policy dimensions of combatting IFFs.
Source: UNCTAD and UNODC.
There is a growing political momentum to measure and monitor sustainable development more effectively and strengthen domestic resource mobilisation. 2025 is a crucial year to strengthen global dialogue and commitment at the FfD44th International Conference of Financing for Development. Countries are increasingly recognizing the high returns on data investment — estimated at an average of $32 for every $1 invested in strengthening data systems in developing economies -—
– ‒
- –
—--—
– ‒
- –
—--—
– ‒
- –
—--—
– ‒
- –
—- — and the significant progress many countries have made in enhancing their national statistical systems. However, despite this momentum, investment in data remains insufficient, and as illicit finance continues to leak out of countries, including in the form of undervalued domestic natural resources, developing countries are seeking solutions to weak development financing. Efforts to track and recover resources lost to IFFs will be a central element in this discussion.
Notes
- Conceptual framework for the statistical measurement of illicit financial flows -—
– ‒
- –
—--—
– ‒
- –
—--—
– ‒
- –
—--—
– ‒
- –
—-; Methodological guidelines to measure tax and commercial illicit financial flows -—
– ‒
- –
—--—
– ‒
- –
—--—
– ‒
- –
—--—
– ‒
- –
—-. - Selecting data series to reflect SDG indicator 16.4.1 on SDG Indicators Database: https://unstats.un.org/sdgs/dataportal/database returns Indicator 16.4.1 series: Total value of inward illicit financial flows (DI_ILL_IN) and Total value of outward illicit financial flows (DI_ILL_OUT).
- Report from the Joint Measurement and Policy Workshop on IFFs, https://unctad.org/system/files/information-document/20250203- 07_measurementpolicyworkshop_finalreport.pdf
- Project “Measuring and curbing illicit financial flows”, 2023-2026, https://unctad.org/project/measuring-and-curbing-illicit-financial-flows
- Project “Statistical measurement of illicit financial flows to enable more targeted policy action”, 2024-2026, https://unctad.org/project/statistical-measurement-tax-and-commercial-illicit-financial-flows-enable-more-targeted.
- Joint Measurement and Policy Workshop on IFFs, https://unctad.org/meeting/joint-measurement-and-policy-workshop-illicit-financial-flows
References
Donec tincidunt vel mauris a dignissim. Curabitur sodales nunc id vestibulum tempor. Nunc tortor orci, sodales nec eros eget.
Donec tincidunt vel mauris a dignissim. Curabitur sodales nunc id vestibulum tempor. Nunc tortor orci, sodales nec eros eget.
Donec tincidunt vel mauris a dignissim. Curabitur sodales nunc id vestibulum tempor. Nunc tortor orci, sodales nec eros eget.
Donec tincidunt vel mauris a dignissim. Curabitur sodales nunc id vestibulum tempor. Nunc tortor orci, sodales nec eros eget.
Donec tincidunt vel mauris a dignissim. Curabitur sodales nunc id vestibulum tempor. Nunc tortor orci, sodales nec eros eget.